Center for Advanced Electronics Through Machine Learning (CAEML)
April 07, 2021
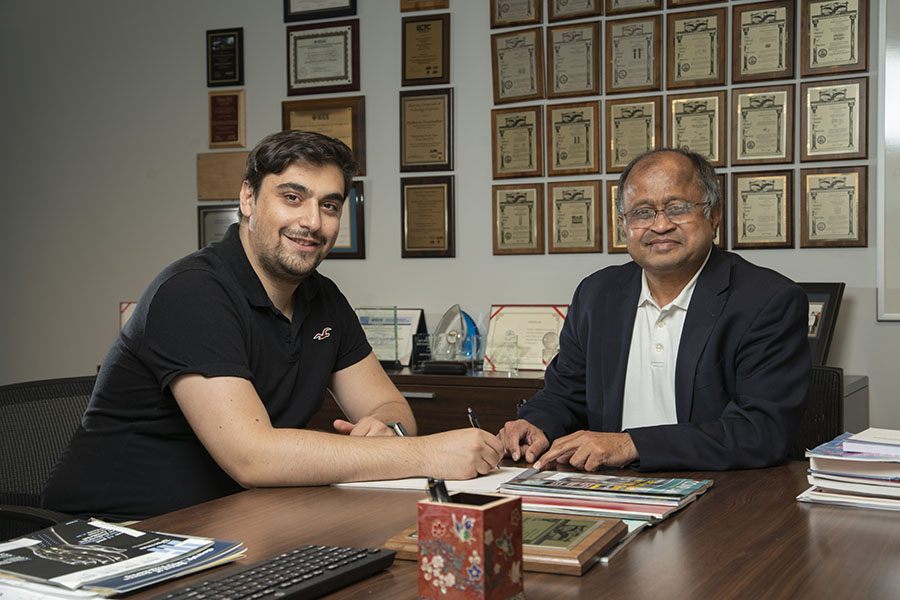
Electronics Development Speeds Up with New Optimization System
Electronics have permeated nearly every part of our lives from medicine to entertainment to the way we work. As the uses of electronics increases, so does the need for highly specialized electronic components. Now, a new method for optimizing electronics has been developed that will dramatically cut the time it takes to get new components and systems to market.
Traditionally, electric components and systems, such as semiconductors and chips, are tuned and tested over months before they are optimized for a task. A new method — which is now available to companies as a software program — uses a statistical technique based on probabilities called Bayesian optimization to replace the usual trial-and-error method.
The new research comes at a time when manufacturing of electronic components is steadily rising. In 2020, the worldwide semiconductor market even grew 5.4% over the previous year, despite the dampening effects of COVID on global economies, and is projected to grow 10.9% in 2021. The United States, a global leader in semiconductor manufacturing, saw $193 billion in sales of semiconductors in 2019 and there is interest in possible manufacturing incentives and research initiatives that could increase the size of the future market.
Statistics for Success
Through a series of iterations, the software is able to statistically determine the exact settings, such as voltage, operating temperature, and material properties, that will optimize a component for a certain use. The new software can easily deal with complex systems whereas previous algorithms may have selected the wrong settings due to incorrect decisions.
Since the new method can almost instantaneously determine optimum settings, it can save developers several months of work that would otherwise be spent testing prototypes.
“In the past, the amount of time from when you start designing a system to the point where you can build something can be very, very long -- six months to a year,” said Madhavan Swaminathan, researcher and professor at the Georgia Tech School of Electrical and Computer Engineering, who headed the project. “The new software program reduces the number of times you have to prototype something before you actually get it right.”
The software was developed by Swaminathan, his graduate students and his collaborators through the Center for Advanced Electronics through Machine Learning (CAEML), one of the National Science Foundation-funded Industry-University Cooperative Research Centers (IUCRC), which enables cutting-edge research on emerging technologies to benefit manufacturing sectors.
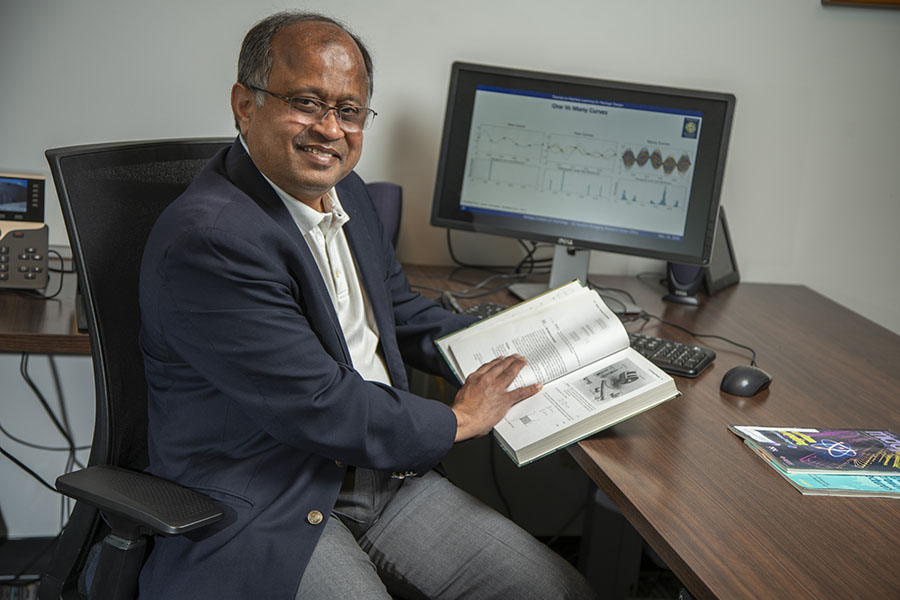
Optimizing for Complex Systems
To optimize systems, the software is also able to compute what’s known as second order interactions. This helps optimize independent settings for elements that influence each other. For example, a semiconductor might be best optimized at a large voltage, but this setting could make the operating temperature be too high, ultimately reducing the performance of the semiconductor chip. The new software is able to take this interdependence into account to find the best settings that optimize the whole system. Previous algorithms were only able to do this in simple cases. The software is also able to compute settings for systems with constraints, such as if there needs to be a limit on the voltage.
Since some systems can be highly complex with multiple components and limitations, the researchers developed three new techniques called Two Stage Bayesian Optimization, Deep-Partitioning Tree Bayesian Optimization and Bayesian Active Learning with Drop Out. This allows optimization to be performed even in deeply interconnected systems with many parameters.
Enormous Applications
The project was done in collaboration with industry partners who are already using the new software to design new electronics systems and improve their workflows.
“Several of the methods developed by CAEML helped accelerate package design optimization flow for high-speed signaling by more than a thousand times,” said Kemal Aygun, Senior Principal Engineer at Intel who collaborated on the project. “This helped significantly reduce the months required for completing these complex designs.”
IBM, who also collaborated with CAEML, has started using the new software to quickly alter high-speed channel design 30 times faster than using traditional methods. “The Bayesian Optimization methods have made our team more efficient in the highly computer-intensive analyses we use to produce IBM systems,” said Wiren Becker, Senior Technical Staff Member at IBM. “We are identifying applications and integrating these methods into our High-Performance Computing cloud infrastructure where we have demonstrated 100 times speed up for some applications in this environment.”
The researchers are continuing to extend the applications of the software through a collaboration with other companies such as Toyota that is focused on designing better inverters for electric vehicles.
“The applications are enormous because no matter where you go, optimization is a very important step before designs go into manufacturing,” Swaminathan said.